Xu Wu
Assistant Professor of Nuclear Engineering
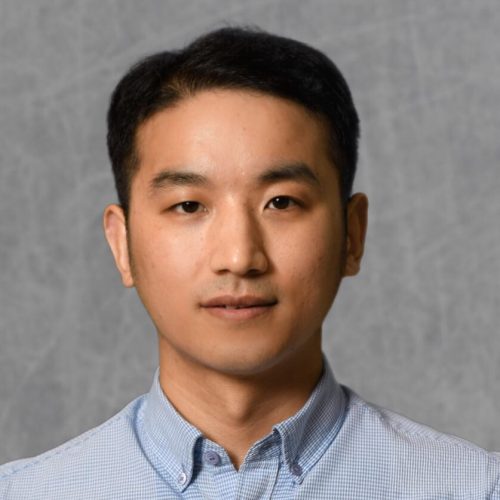
- 919-515-6570
- xwu27@ncsu.edu
- Burlington Laboratory 2110
- Visit My Website
- View CV
Dr. Wu received his B.S. degree in nuclear engineering from the Shanghai Jiao Tong University in China in 2011. He obtained his Ph.D. degree in nuclear engineering in 2017 from the University of Illinois at Urbana – Champaign. Prior to joining NCSU, he was a Postdoctoral Research Associate in the Department of Nuclear Science and Engineering at Massachusetts Institute of Technology. Dr. Wu is the principal investigator (PI) of the ARTISANS (ARTificial Intelligence for Simulation of Advanced Nuclear Systems) research group at NCSU-NE. The research in the ARTISANS group revolves around uncertainty quantification (UQ) and scientific machine learning (SciML). The goal is to combine SciML, experimentation and modeling & simulation (M&S) into a unified approach to improve the predictive capabilities of computer models and enable reduced reliance on expensive measurement data. Additionally, the application of such research will be focused on risk and economics evaluations of advanced nuclear reactors, such as small modular reactors and micro-reactors. The ultimate goal is to dramatically reduce the capital and operating costs of nuclear systems to maintain global technology leadership for nuclear energy.
Education
Nuclear Engineering
Shanghai Jiao Tong University
Nuclear, Plasma and Radiological Engineering
University of Illinois at Urbana - Champaign
Nuclear, Plasma and Radiological Engineering
University of Illinois at Urbana - Champaign
Nuclear Science and Engineering
Massachusetts Institute of Technology
Research Description
Dr. Wu's research interests include: (1) calibration, validation, data assimilation, uncertainty and sensitivity analysis; (2) computational statistics, reduced order modeling; (3) Bayesian inverse problems; (4) scientific machine learning and deep generative learning; (5) system thermal-hydraulics, nuclear fuel performance modeling, multi-physics coupled simulation; (6) advanced reactors, small modular reactors, micro-reactors.
Publications
- Data-driven prediction and uncertainty quantification of PWR crud-induced power shift using convolutional neural networks
- Furlong, A., Alsafadi, F., Palmtag, S., Godfrey, A., & Wu, X. (2025), ENERGY, 316. https://doi.org/10.1016/j.energy.2025.134447
- Sensitivity and uncertainty analysis in pebble-bed reactors: A study using the High-Temperature Code Package (HCP)
- Yaseen, M., Sadek, A., Osman, W., Altahhan, M., Wu, X., Avramova, M., & Ivanov, K. (2025), ANNALS OF NUCLEAR ENERGY, 219. https://doi.org/10.1016/j.anucene.2025.111428
- A systematic approach for the adequacy analysis of a set of experimental databases: Application in the framework of the ATRIUM activity
- Baccou, J., Glantz, T., Ghione, A., Sargentini, L., Fillion, P., Damblin, G., … Adorni, M. (2024), NUCLEAR ENGINEERING AND DESIGN, 421. https://doi.org/10.1016/j.nucengdes.2024.113035
- ARTISANS-Artificial Intelligence for Simulation of Advanced Nuclear Systems for Nuclear Fission Technology
- Akins, A., Furlong, A., Kohler, L., Clifford, J., Brady, C., Alsafadi, F., & Wu, X. (2024), NUCLEAR ENGINEERING AND DESIGN, 423. https://doi.org/10.1016/j.nucengdes.2024.113170
- Clustering and uncertainty analysis to improve the machine learning-based predictions of SAFARI-1 control follower assembly axial neutron flux profiles
- Moloko, L. E., Bokov, P. M., Wu, X., & Ivanov, K. N. (2024), ANNALS OF NUCLEAR ENERGY, 206. https://doi.org/10.1016/j.anucene.2024.110630
- Design considerations and Monte Carlo criticality analysis of spiral plate heat for Molten Salt Reactors
- Brady, C., Murray, W., Moss, L., Zino, J., Saito, E., & Wu, X. (2024), PROGRESS IN NUCLEAR ENERGY, 173. https://doi.org/10.1016/j.pnucene.2024.105266
- Functional PCA and deep neural networks-based Bayesian inverse uncertainty quantification with transient experimental data
- Xie, Z., Yaseen, M., & Wu, X. (2024), COMPUTER METHODS IN APPLIED MECHANICS AND ENGINEERING, 420. https://doi.org/10.1016/j.cma.2023.116721
- Hierarchical Bayesian modeling for Inverse Uncertainty Quantification of system thermal-hydraulics code using critical flow experimental data
- Xie, Z., Wang, C., & Wu, X. (2025), INTERNATIONAL JOURNAL OF HEAT AND MASS TRANSFER, 239. https://doi.org/10.1016/j.ijheatmasstransfer.2024.126489
- Deep generative modeling-based data augmentation with demonstration using the BFBT benchmark void fraction datasets
- Alsafadi, F., & Wu, X. (2023), NUCLEAR ENGINEERING AND DESIGN, 415. https://doi.org/10.1016/j.nucengdes.2023.112712
- Fast and accurate reduced-order modeling of a MOOSE-based additive manufacturing model with operator learning
- Yaseen, M., Yushu, D., German, P., & Wu, X. (2023), INTERNATIONAL JOURNAL OF ADVANCED MANUFACTURING TECHNOLOGY, 129(7-8), 3123–3139. https://doi.org/10.1007/s00170-023-12471-1